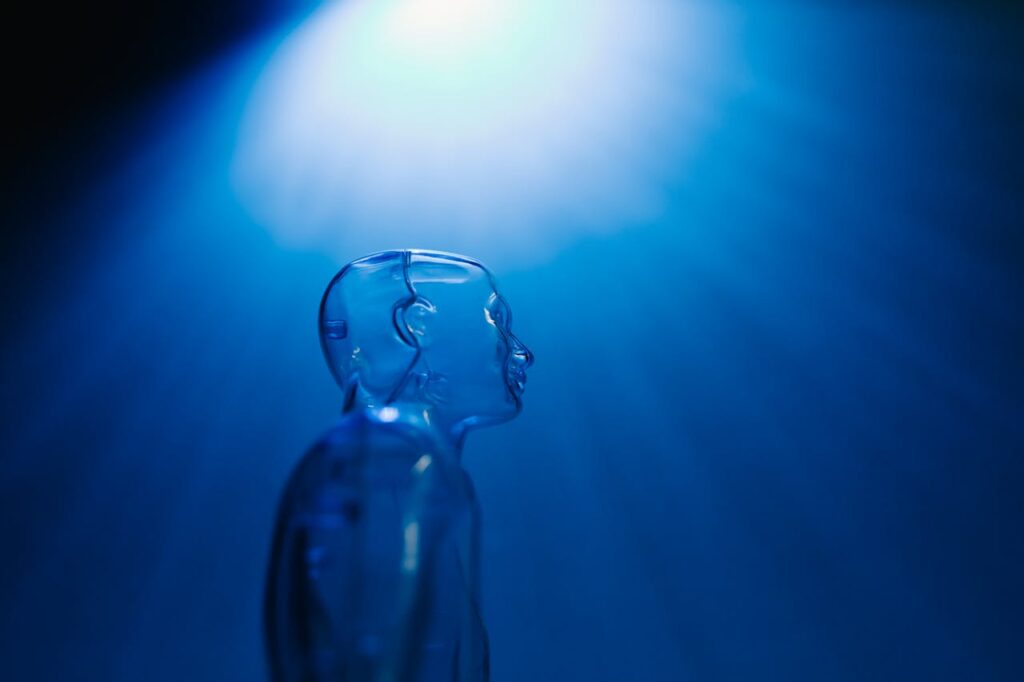
Introduction
AI Implementation Challenges in Large Companies encompass a range of complex issues that organizations must navigate to successfully integrate artificial intelligence into their operations. While AI presents tremendous opportunities for enhancing efficiency, productivity, and innovation, large companies often face significant hurdles during the implementation phase. These challenges include data complexity, technological infrastructure, skills shortages, ethical and legal issues, resistance to change, and high costs. This article explores each of these challenges in depth and offers strategies for overcoming them.
1. Data Complexity in AI Implementation
AI Implementation Challenges in Large Companies frequently begin with managing and utilizing complex data. For AI systems to function effectively, they rely on large volumes of high-quality, diverse data. In large organizations, data often comes from numerous sources, including customer interactions, operational processes, and market analysis. Ensuring that this data is accurate, comprehensive, and integrated can be a significant challenge.
Challenges:
- Data Silos: In many large companies, data is stored in separate systems across different departments or business units. This fragmentation can hinder the integration and analysis of data, making it difficult to obtain a unified view necessary for AI applications.
- Data Quality: The effectiveness of AI models heavily depends on the quality of the data they use. Poor-quality or incomplete data can lead to inaccurate predictions, biased results, and suboptimal decision-making.
Strategies:
- Data Governance: Implement a robust data governance framework to oversee data quality, management, and integration. Establish clear protocols for data collection, storage, and usage to ensure consistency and accuracy.
- Integration Tools: Use advanced data integration tools such as data lakes or ETL platforms to consolidate data from various sources. These tools can help create a unified data repository, making it easier for AI systems to access and analyze the information.
Examples:
- Retailers: A large retail chain may struggle with integrating data from online sales, in-store transactions, and inventory systems. Effective integration can enhance personalized marketing efforts and optimize inventory management.
- Manufacturers: Integrating production data from multiple facilities can improve supply chain efficiency and predictive maintenance.
2. Technological Infrastructure Challenges
Implementing AI requires a robust technological infrastructure capable of supporting AI applications. Large companies may need to update or overhaul their existing systems to meet the demands of AI technologies, which often require substantial computing power and storage capacity.
Challenges:
- Performance Requirements: AI models, particularly those involving deep learning, require high-performance computing resources. Traditional infrastructure may not be sufficient to handle the intensive processing demands of AI applications.
- Scalability: As AI projects scale, the underlying infrastructure must be able to accommodate increasing data volumes and computational needs. This requires a scalable and flexible technology stack.
Strategies:
- Cloud Solutions: Leverage cloud computing services such as Amazon Web Services (AWS), Google Cloud Platform (GCP), or Microsoft Azure. These platforms offer scalable and flexible resources, allowing companies to adjust their computing power and storage as needed.
- Infrastructure Investment: Invest in high-performance servers, GPUs (Graphics Processing Units), and specialized hardware designed for AI workloads. Ensure that your infrastructure can scale with the growing demands of AI projects.
Examples:
- Financial Services: Banks and financial institutions may need to upgrade their IT infrastructure to support real-time AI-driven fraud detection and risk management systems.
- Healthcare: Hospitals might require enhanced computing power for processing large datasets used in medical imaging and diagnostics.
3. Skills and Expertise Shortages
The shortage of skilled professionals is a major AI Implementation Challenge in Large Companies. Implementing AI effectively requires expertise in data science, machine learning, and project management, among other areas. Finding or developing these skills within an organization can be difficult.
Challenges:
- Talent Scarcity: The demand for AI specialists often exceeds the supply. Finding qualified candidates with the right skills and experience can be a significant challenge.
- Skill Gaps: Existing employees may lack the advanced skills needed for AI development and implementation. This skills gap can hinder the successful deployment of AI technologies.
Strategies:
- Training Programs: Develop in-house training programs or collaborate with educational institutions to upskill employees. Offering courses and workshops in data science, machine learning, and AI can help bridge the skills gap.
- Hiring Experts: Recruit external consultants or specialists with a proven track record in AI to guide and support AI initiatives. Consider forming partnerships with AI research institutions or consulting firms to access specialized expertise.
Examples:
- Tech Giants: Companies like Google and Microsoft invest heavily in recruiting top AI talent to maintain their competitive edge and drive innovation.
- Startups: Smaller companies may collaborate with universities or AI research centers to access cutting-edge expertise and technology.
4. Ethical and Legal Issues
AI Implementation Challenges in Large Companies also involve addressing ethical and legal concerns. AI technologies raise important issues related to privacy, data protection, and fairness. Ensuring that AI systems comply with regulations and do not perpetuate biases is crucial for maintaining trust and legality.
Challenges:
- Privacy Concerns: AI systems often process sensitive personal data, raising concerns about data privacy and compliance with regulations such as the General Data Protection Regulation (GDPR).
- Bias and Fairness: AI systems can unintentionally perpetuate biases present in the data they are trained on, leading to discriminatory outcomes. Ensuring fairness and avoiding bias in AI decision-making is a critical challenge.
Strategies:
- Ethical Guidelines: Develop and adhere to ethical guidelines for AI development and deployment. Focus on transparency, accountability, and fairness in AI systems to address ethical concerns.
- Regular Audits: Conduct regular audits of AI systems to assess compliance with legal standards and ethical practices. Implement mechanisms to detect and mitigate biases in AI algorithms.
Examples:
- Social Media: Platforms like Facebook and Twitter must ensure that their AI-driven content moderation systems do not unfairly censor or discriminate against users.
- Hiring Algorithms: Companies using AI in recruitment must carefully monitor algorithms to prevent biases that could affect the fairness of hiring decisions.
5. Resistance to Change
Resistance to change is a common AI Implementation Challenge in Large Companies. Employees and management may be apprehensive about the impact of AI on job roles and organizational processes. Managing this resistance is essential for successful AI adoption.
Challenges:
- Employee Concerns: Employees may fear job loss or changes to their roles due to AI automation. Addressing these concerns is crucial for maintaining morale and engagement.
- Change Management: Effectively managing the transition to AI-driven processes requires clear communication and support for employees throughout the change process.
Strategies:
- Communication: Clearly communicate the benefits of AI and how it will enhance rather than replace human roles. Highlight how AI can improve job conditions and create new opportunities.
- Support Programs: Implement support programs, including workshops and training sessions, to help employees adapt to new technologies and roles. Offer resources and support to ease the transition.
Examples:
- Manufacturing: Workers in manufacturing plants may resist automation if they perceive it as a threat to their jobs. Clear communication about the benefits of automation and new roles can help mitigate resistance.
- Customer Service: AI-driven chatbots might face resistance from customer service representatives concerned about job displacement. Providing training and support can help employees adapt to new roles.
6. High Costs of AI Projects
The financial investment required for AI projects is a significant AI Implementation Challenge in Large Companies. Implementing AI involves substantial costs for technology, training, and infrastructure, which can be a barrier to adoption.
Challenges:
- Upfront Investment: The initial costs for AI technology, including hardware, software, and implementation, can be high. This investment may be a significant hurdle for large companies.
- ROI Uncertainty: Achieving a positive return on investment (ROI) from AI projects may not be immediate, making financial planning and management critical.
Strategies:
- Phased Implementation: Start with pilot projects to demonstrate the value of AI before committing to a full-scale deployment. This approach allows companies to test and refine AI technologies while managing costs.
- Budget Planning: Develop a detailed budget that covers all potential costs associated with AI projects, including long-term maintenance and support. Plan for ongoing investments in technology and talent.
Examples:
- Retail Chains: A retail chain might initiate a pilot project for AI-driven inventory management before expanding the technology across all locations.
- Healthcare Providers: Hospitals may begin with AI tools for diagnostics in specific departments before scaling the technology organization-wide.
Conclusion
Navigating AI Implementation Challenges in Large Companies requires addressing a range of issues, including data complexity, infrastructure needs, skills shortages, ethical and legal concerns, resistance to change, and high costs. By developing strategies to overcome these challenges, large organizations can harness the full potential of AI, driving innovation and achieving long-term success in a rapidly evolving market. Effective management of these challenges will enable companies to integrate
Stay tuned to WebVibe’s blog for more insights on digital marketing and SEO strategies!